Utilities for applying scikit-learn to spatial datasets
Project description

## Python module for geospatial prediction using scikit-learn and rasterio
`pyimpute` provides high-level python functions for bridging the gap between spatial data formats and machine learning software to facilitate supervised classification and regression on geospatial data. This allows you to create landscape-scale predictions based on sparse observations.
The observations, known as the **training data**, consists of:
* response variables: what we are trying to predict
* explanatory variables: variables which explain the spatial patterns of responses
The **target data** consists of explanatory variables represented by raster datasets. There are no response variables available for the target data; the goal is to *predict* a raster surface of responses. The responses can either be discrete (classification) or continuous (regression).
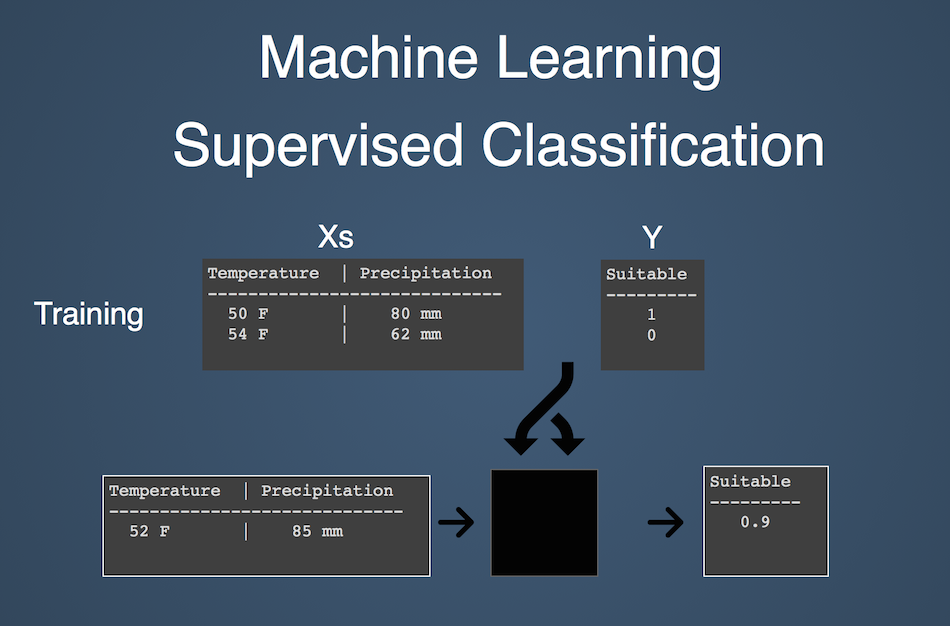
## Pyimpute Functions
* `load_training_vector`: Load training data where responses are vector data (explanatory variables are always raster)
* `load_training_raster`: Load training data where responses are raster data
* `stratified_sample_raster`: Random sampling of raster cells based on discrete classes
* `evaluate_clf`: Performs cross-validation and prints metrics to help tune your scikit-learn classifiers.
* `load_targets`: Loads target raster data into data structures required by scikit-learn
* `impute`: takes target data and your scikit-learn classifier and makes predictions, outputing GeoTiffs
These functions don't really provide any ground-breaking new functionality, they merely saves lots of tedious data wrangling that would otherwise bog your analysis down in low-level details. In other words, `pyimpute` provides a high-level python workflow for spatial prediction, making it easier to:
* explore new variables more easily
* frequently update predictions with new information (e.g. new Landsat imagery as it becomes available)
* bring the technique to other disciplines and geographies
### Basic example
Here's what a `pyimpute` workflow might look like. In this example, we have two explanatory variables as rasters (temperature and precipitation) and a geojson with point observations of habitat suitability for a plant species. Our goal is to predict habitat suitability across the entire region based only on the explanatory variables.
```
from pyimpute import load_training_vector, load_targets, impute, evaluate_clf
from sklearn.ensemble import RandomForestClassifier
```
Load some training data
```
explanatory_rasters = ['temperature.tif', 'precipitation.tif']
response_data = 'point_observations.geojson'
train_xs, train_y = load_training_vector(response_data,
explanatory_rasters,
response_field="suitability")
```
Train a scikit-learn classifier
```
clf = RandomForestClassifier(n_estimators=10, n_jobs=1)
clf.fit(train_xs, train_y)
```
Evalute the classifier using several validation metrics, manually inspecting the output
```
evaluate_clf(clf, train_xs, train_y)
```
Load target raster data
```
target_xs, raster_info = load_targets(explanatory_rasters)
```
Make predictions, outputing geotiffs
```
impute(target_xs, clf, raster_info, outdir='/tmp',
linechunk=400, class_prob=True, certainty=True)
assert os.path.exists("/tmp/responses.tif")
assert os.path.exists("/tmp/certainty.tif")
assert os.path.exists("/tmp/probability_0.tif")
assert os.path.exists("/tmp/probability_1.tif")
```
### Installation
Assuming you have `libgdal` and the scipy system dependencies installed, you can install with pip
```
pip install pyimpute
```
Alternatively, install from the source code
```
git clone https://github.com/perrygeo/pyimpute.git
cd pyimpute
pip install -e .
```
See the `.travis.yml` file for a working example on Ubuntu systems.
### Other resources
For an overview, watch my presentation at FOSS4G 2014: <a href="http://vimeo.com/106235287">Spatial-Temporal Prediction of Climate Change Impacts using pyimpute, scikit-learn and GDAL — Matthew Perry</a>
Also, check out [the examples](https://github.com/perrygeo/python-impute/blob/master/examples/) and [the wiki](https://github.com/perrygeo/pyimpute/wiki)
## Python module for geospatial prediction using scikit-learn and rasterio
`pyimpute` provides high-level python functions for bridging the gap between spatial data formats and machine learning software to facilitate supervised classification and regression on geospatial data. This allows you to create landscape-scale predictions based on sparse observations.
The observations, known as the **training data**, consists of:
* response variables: what we are trying to predict
* explanatory variables: variables which explain the spatial patterns of responses
The **target data** consists of explanatory variables represented by raster datasets. There are no response variables available for the target data; the goal is to *predict* a raster surface of responses. The responses can either be discrete (classification) or continuous (regression).
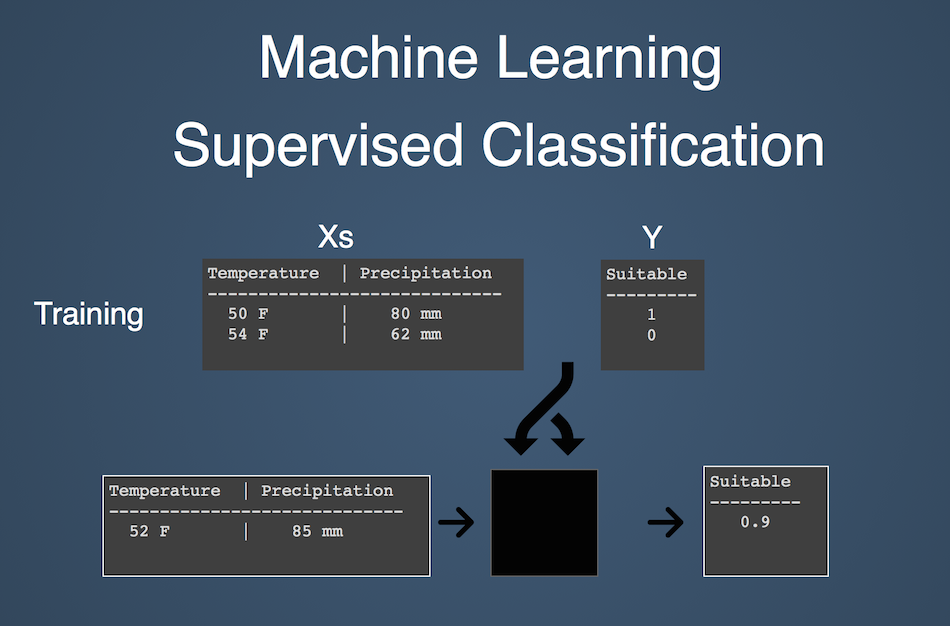
## Pyimpute Functions
* `load_training_vector`: Load training data where responses are vector data (explanatory variables are always raster)
* `load_training_raster`: Load training data where responses are raster data
* `stratified_sample_raster`: Random sampling of raster cells based on discrete classes
* `evaluate_clf`: Performs cross-validation and prints metrics to help tune your scikit-learn classifiers.
* `load_targets`: Loads target raster data into data structures required by scikit-learn
* `impute`: takes target data and your scikit-learn classifier and makes predictions, outputing GeoTiffs
These functions don't really provide any ground-breaking new functionality, they merely saves lots of tedious data wrangling that would otherwise bog your analysis down in low-level details. In other words, `pyimpute` provides a high-level python workflow for spatial prediction, making it easier to:
* explore new variables more easily
* frequently update predictions with new information (e.g. new Landsat imagery as it becomes available)
* bring the technique to other disciplines and geographies
### Basic example
Here's what a `pyimpute` workflow might look like. In this example, we have two explanatory variables as rasters (temperature and precipitation) and a geojson with point observations of habitat suitability for a plant species. Our goal is to predict habitat suitability across the entire region based only on the explanatory variables.
```
from pyimpute import load_training_vector, load_targets, impute, evaluate_clf
from sklearn.ensemble import RandomForestClassifier
```
Load some training data
```
explanatory_rasters = ['temperature.tif', 'precipitation.tif']
response_data = 'point_observations.geojson'
train_xs, train_y = load_training_vector(response_data,
explanatory_rasters,
response_field="suitability")
```
Train a scikit-learn classifier
```
clf = RandomForestClassifier(n_estimators=10, n_jobs=1)
clf.fit(train_xs, train_y)
```
Evalute the classifier using several validation metrics, manually inspecting the output
```
evaluate_clf(clf, train_xs, train_y)
```
Load target raster data
```
target_xs, raster_info = load_targets(explanatory_rasters)
```
Make predictions, outputing geotiffs
```
impute(target_xs, clf, raster_info, outdir='/tmp',
linechunk=400, class_prob=True, certainty=True)
assert os.path.exists("/tmp/responses.tif")
assert os.path.exists("/tmp/certainty.tif")
assert os.path.exists("/tmp/probability_0.tif")
assert os.path.exists("/tmp/probability_1.tif")
```
### Installation
Assuming you have `libgdal` and the scipy system dependencies installed, you can install with pip
```
pip install pyimpute
```
Alternatively, install from the source code
```
git clone https://github.com/perrygeo/pyimpute.git
cd pyimpute
pip install -e .
```
See the `.travis.yml` file for a working example on Ubuntu systems.
### Other resources
For an overview, watch my presentation at FOSS4G 2014: <a href="http://vimeo.com/106235287">Spatial-Temporal Prediction of Climate Change Impacts using pyimpute, scikit-learn and GDAL — Matthew Perry</a>
Also, check out [the examples](https://github.com/perrygeo/python-impute/blob/master/examples/) and [the wiki](https://github.com/perrygeo/pyimpute/wiki)
Project details
Download files
Download the file for your platform. If you're not sure which to choose, learn more about installing packages.
Source Distributions
pyimpute-0.1.1.zip
(13.5 kB
view details)
pyimpute-0.1.1.tar.gz
(7.7 kB
view details)
File details
Details for the file pyimpute-0.1.1.zip
.
File metadata
- Download URL: pyimpute-0.1.1.zip
- Upload date:
- Size: 13.5 kB
- Tags: Source
- Uploaded using Trusted Publishing? No
File hashes
Algorithm | Hash digest | |
---|---|---|
SHA256 | 8750a773232c321b06bac17f7ae8694997f9db8c08ca022e86b8d272d092287e |
|
MD5 | 7a50dcf61f4bdf59280083c620ace3f8 |
|
BLAKE2b-256 | fc314ee80da7dee442fcad2dbf1b5aa3a0a71eb63eae9b5885143228f6149c56 |
File details
Details for the file pyimpute-0.1.1.tar.gz
.
File metadata
- Download URL: pyimpute-0.1.1.tar.gz
- Upload date:
- Size: 7.7 kB
- Tags: Source
- Uploaded using Trusted Publishing? No
File hashes
Algorithm | Hash digest | |
---|---|---|
SHA256 | 53177765c367b34fd02add81530ad5a58c635951647740755c59683dca5d9e50 |
|
MD5 | 6c6ae8e47034a7509458406528d30224 |
|
BLAKE2b-256 | 99c62df42b5bb58aaa4df884324408e0bf568dec4514ac10e225a1982bc550cf |